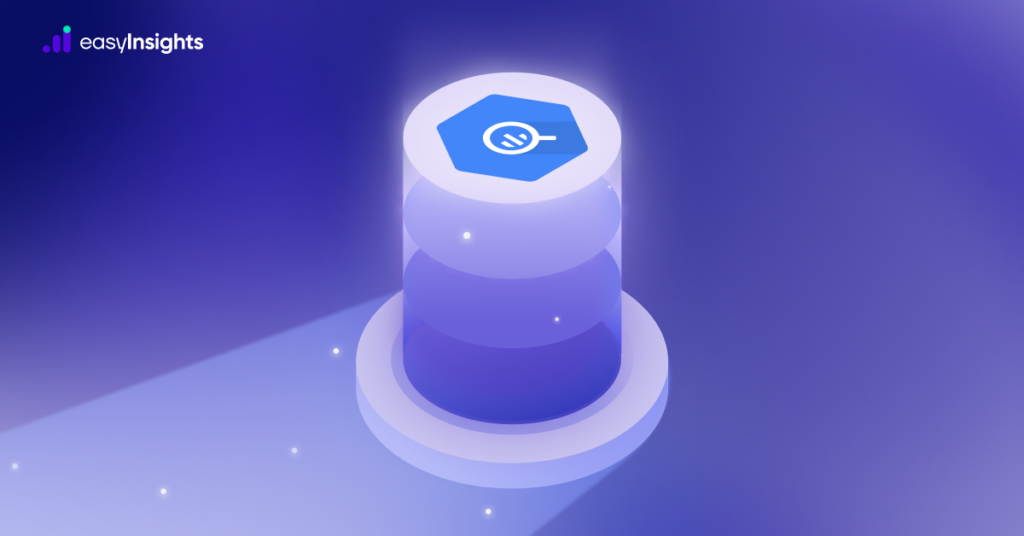
Tools like spreadsheets work great if you want to create pivot tables, build basic calculations, and visualize your data. But as your data volume increases, and your data analysis needs become more complex, you must upgrade to a more comprehensive tool such as Google BigQuery.
After it was launched in 2010, Google BigQuery has become one of the leading data warehouses for marketers. Capable of running SQL queries on terabytes of data in just a few moments, this Google product is exactly what data-driven marketing teams need.
Here we give you a complete overview of Google BigQuery, including how you can integrate it into your marketing plans to drive efficiency.
Jump ahead to:
What is Google BigQuery?
BigQuery is a fully managed serverless, scalable data warehouse that operates on Google Cloud. This Google offering allows you to upload massive data sets, and explore them with ease. Not only that, it also features Machine Learning (ML) capabilities to help process data in dynamic ways.
Further, it allows you to conduct batch queries to create tables from collated data. BigQuery also generates data reports to help analyze huge datasets quickly. Lastly, it fosters collaboration among marketing teams by empowering users to share spreadsheets, reports, queries, and the like seamlessly.
The BigQuery Sandbox
BigQuery is a comprehensive Google offering that comes with a staggering number of features. And it’s only natural if marketers hesitate to explore all these for in fear they might cost them steeply. For them, we have two words- BigQuery Sandbox.
This free, completely functioning version of Google BigQuery allows you to experiment and navigate BigQuery’s offerings without the price tag. So, you can explore this tool without sharing your credit card details and judge whether it’s a good fit for you. With BigQuery sandbox, you’ll have access to storage, queries, data processing capabilities, and other resources, but for a limited time.
Why should Marketers consider Google BigQuery?
Here are some of BigQuery’s notable benefits that make it an essential tool for marketers today.
- Higher Data Storage and Processing Capacity
Alternative tools such as Microsoft Excel and Google Sheets have limited data storage and analysis capabilities. However, BigQuery doesn’t suffer from this shortcoming. It is specially designed to hold massive amounts of data while also offering functions to explore it.
In other words, you can transfer all your marketing data onto one centralized platform, without having to worry about data volume.
- Retains Historical Data
A significant shortcoming of regular data analysis tools is that they’re unable to keep all data in one place. That is to say, you can explore fresh data, but if you want to cross-examine it with past datasets, you might hit a wall.
And so, another reason to integrate BigQuery into your operations is its ability to retain historical data. Marketers can revisit older data to compare present performance with the past.
- Offers Granular View of Data
BigQuery empowers you to dig into your data the way you want to. While every marketing platform will have its set of metrics you can access, with BigQuery you have no such limitation. It offers data granularity that you can’t access on native interfaces. So, if you want to get to every last data point in your data set, BigQuery can help.
- Offers Ease of Implementation
BigQuery is a cheaper and easier alternative to setting up an on-premise data warehouse. While you’ll have to build infrastructure from scratch with a native solution, BigQuery allows you to access a full-fledged data warehousing platform over the cloud.
Being a cloud platform, it is highly scalable based on your evolving needs for data. Further, with Google BigQuery, you only pay for what you use, making it highly economical.
- Processes Data at Lightning Speed
BigQuery processes an impressive amount of data in a matter of seconds. And so, if you’re looking for real-time information on your campaign performance, BigQuery has you covered.
- Columnar Database
BigQuery uses a columnar storage structure or a column-based storage system to help it optimize query processing with limited resources. This structure plays a key role in enabling BigQuery to handle massive datasets without compromising on speed.
Storing data in columns as opposed to rows allows quicker and more thorough reading of data. This is especially true when data is stored for analytical purposes. On the other hand, row-based storage is better suited for transactional databases.
- SQL and Programming Language Support
BigQuery allows users to access its architecture with standard SQL. However, for users who prefer Python, Java, PHP, Ruby, C#, or Node.js, BigQuery offers client libraries to assist them as well.
- Serverless Service
Data warehouses usually rely on server hardware to run computations. However, this requires administrators to provide data elasticity, reliability, and security among many things. Fortunately, BigQuery functions on a serverless model that removes this constraint altogether. Now, data administrators can focus on extracting insights rather than on the infrastructure.
- Real-time Analytics
BigQuery doesn’t just process stored data but can help you analyze real-time data as well. You can extract insights from data stored in your warehouse using other Google Cloud Platform (GCP) services and tools such as Looker Studio and Google Analytics.
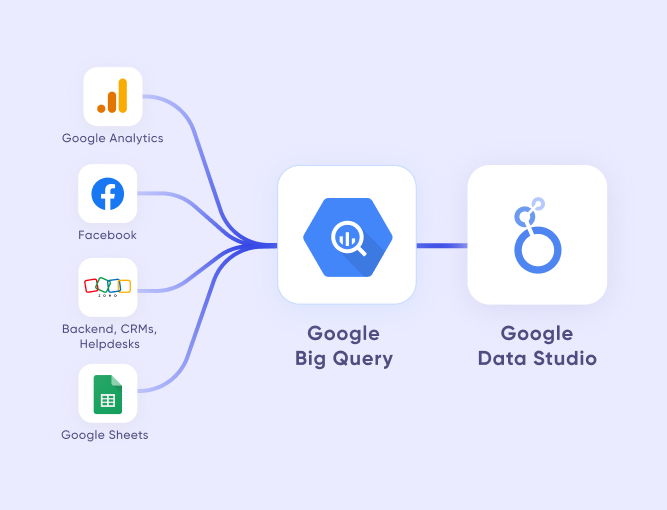
Data in Google BigQuery: Explained
Working with data in BigQuery requires users to take certain specific steps. Here’s a look at how to navigate this Google platform.
- Data Upload
Users can upload data from a variety of sources such as Google Cloud Storage, CSV files, and JSON files. Irrespective of whether you’re using its web UI, APIs, or command-line tools, BigQuery allows you to load up data from multiple sources.
- Data Modeling
BigQuery doesn’t require you to define a schema beforehand; it uses a schema-on-read approach instead. However, while you don’t have to specify a schema initially, it can be advantageous for query optimization and general performance. You can store data using views, partitions, or tables in BigQuery.
- Data Querying
BigQuery supports standard SQL syntax for data filtering and analysis. Given its intricate structure, BigQuery can process even the vastest datasets in just a few moments.
- Data Transformation
While BigQuery does offer SQL capabilities, users can also connect it with tools such as Cloud Dataflow and Dataprep for data transformation. Once your data is transformed, you can then create fresh tables for the refined data.
- Data Visualization
BigQuery doesn’t offer data visualization capabilities. However, users can integrate tools such as Looker Studio with BigQuery seamlessly.
- Data Export
Once you’re done analyzing data in BigQuery, you can export it to Google Cloud Storage, or even services like Google Drive or Google Sheets. Further, it supports export in various formats such as JSON, Avro, CSV, or even Parquet.
Additional read: GA4 & the Google Marketing Platform – The Collaboration that Strengthens your Digital Marketing Strategy
BigQuery Use Cases for Marketers
Now that you know what BigQuery is, and how it works, it’s time to discuss how it can help your marketing pipeline.
- Drive the Performance of Web Analytics and CRM
Your prospect is likely interacting with a considerable number of your pages online before they convert. Now, during this time their user data will be registered under a unique cookie ID, which will be sent to BigQuery.
Post conversion, they’ll receive a unique CRM record ID which will be associated with their cookie ID before conversion. By collating the data from these two user IDs, markets can create a high-quality customer database and use the information to improve their campaign’s targeting capabilities.
- Attribution Analysis for Cross-Channel PPC Analysis
As a marketer, chances are you’re running ad campaigns on multiple platforms such as Facebook ads, Microsoft ads etc. A common grievance you might be facing is the inability to gain an accurate picture of which platform is performing better. BigQuery tackles this effectively.
With this tool, you can capture conversions from all platforms with timestamps and then tag them to a single ID. Now, you can attribute the conversion correctly depending on the modeling scheme you’ve selected.
Gaining such granular insight helps you understand whether your PPC analysis is accurate, or requires reevaluation.
- Analyze Content Marketing Performance
When it comes to content marketing, analyzing how your efforts are doing can be challenging. After all, you probably have multiple tags on each piece of content- from author and content theme to content format and product features. In other words, generating performance reports to gain answers to the following is no easy task.
- How do your Facebook posts content perform compared to your website content?
- Is your 2023 ad campaign performing better than your 2022 campaign?
Answering these questions requires a significant size of data which BigQuery alternatives cannot support. And so, when it comes to content marketing performance reports, BigQuery’s ability to store massive amounts of data allows you to gain better insight.
- Build Demand Forecasts and Predictive Audiences
BigQuery offers ML capabilities. Couple that with its ability to host massive amounts of data at once and you have the perfect combination to create marketing forecasts that can hold you steady through industry changes.
You can analyze past marketing data to create precise forecasts and, in the process, help you plan your campaign calendar accurately. Not only that, you can also factor in seasonality in the industry, to take complete advantage of business peaks.
Lastly, with BigQuery, you can create predictive audiences as well. For instance, you could analyze the propensity to purchase, or customer lifetime values based on Google Analytics data to help target users most likely to convert.
- Ad Cost Optimization
Another way BigQuery comes in handy is by helping you allocate your ads budget optimally. As a marketer running ads on multiple platforms, you need to know whether your PPC strategy is robust and whether platforms are perhaps underperforming in this department when compared to their resource allocation.
Collect data from Facebook, LinkedIn, Instagram, etc., onto your BigQuery account and create a comprehensive visual report of all your blended data. Doing this allows you to allocate your marketing budgets better and thus improve ROI.
Additional read: Free Raw Data Export From Google Analytics4 (GA4) to Google BigQuery – An Easy Guide
Google Big Query: Best Practices for Marketers
1. Denormalize the Data
Denormalizing data is a technique that adds redundant copies of data to an otherwise normalized database to improve the read performance of a database. BigQuery works best when data is denormalized. So, try increasing the instances of nested and repeated fields to improve your BigQuery experience.
2. Harness The Power of Partitioned Tables
Incorporating partitioned tables reduces your BigQuery cost significantly. This is because when we utilize partitioned tables and group data based on logical grounds such as specific criteria or time periods, it reduces the amount of data BigQuery has to scan to answer a query. This naturally reduces the scanning bytes and by extension the cost of leveraging BigQuery.
3. Consolidate Update Queries
Users must avoid running individual row update queries because BigQuery as a tool is meant to work with massive data sets. Since the entire idea behind this Google offering is to facilitate quicker and better data processing for large datasets, engaging it for small tasks can translate into higher costs. And so, always try to batch your update queries.
Service Limits for Google BigQuery
As powerful as Google BigQuery is, marketers must be aware of its service limits to leverage the tool optimally. Here’s a look at these.
- It doesn’t have a daily query limit; users can make unlimited queries
- It has a daily destination table update limit of 1000 updates per table
- It has a query execution time limit of six hours
- It allows a maximum of 1000 tables to be referenced for a single query
- The maximum unresolved query length is 256 KB
- The maximum resolved query length is 12 MB.
- The concurrent rate limit for on-demand, interactive queries is 50
- The concurrent rate limit for on-demand, interactive queries against Cloud Big table external data sources is 4
Partner with EasyInsights to Drive Google BigQuery Efficiency
Google BigQuery has significant benefits, making it an essential tool for modern marketers looking to scale their campaigns efficiently.
But as convenient as its functions are, BigQuery has a steep learning curve, especially for marketers. That’s why created EasyInsights, a next-gen marketing automation platform.
EasyInsights can sync your first-party data from your single source of truth to over 500+ marketing ad platforms, CRMS, CEPs, and more – be it an application or an analytics tool. With this data, you can create audience segments and send signals to ad platforms to optmise their algorithms.
Bonus Read: How EasyInsights works with Raw GA4 Data for Data Activation and Enrichment
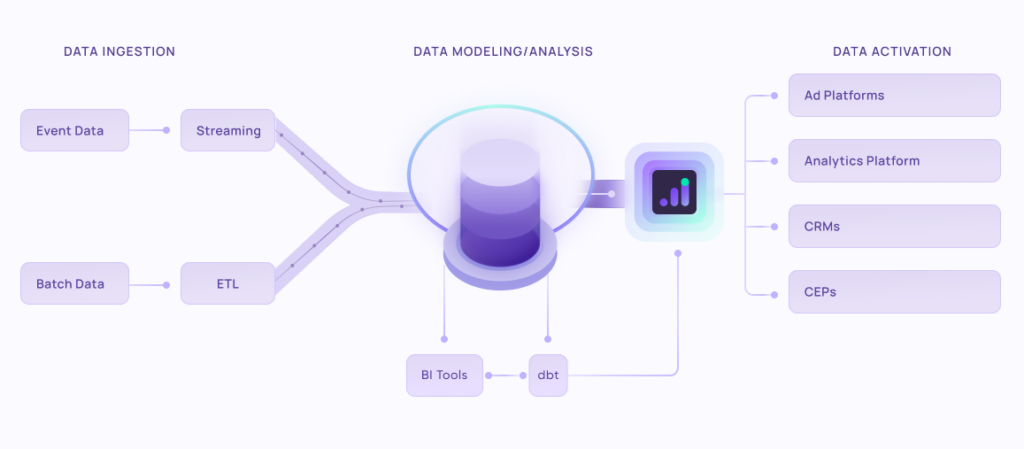
EasyInsights works with raw GA4 data for data activation and data enrichment in a few key ways:
Data Collection and Transformation:
- EasyInsights connect directly to your Google Analytics 4 property allowing it to access and extract the raw GA4 data you’ve collected.
- Data Transformation: EasyInsights then cleans, normalizes, and enriches the data to make it usable for activation purposes. This may involve tasks like:
- Formatting data: Ensuring all data points are in a consistent format.
- Handling missing values: Filling in missing data points or removing them according to your preferences.
- Enriching data: Adding additional data points from other sources to create a more comprehensive picture of your customers.
Data Activation:
- Segmentation and Targeting: It allows you to segment your audience based on various criteria like demographics, behavior, and interests using the enriched GA4 data.
- Multi-channel Marketing: You can then use these segments to activate your audience across different marketing channels like email, social media, and advertising platforms.
- Customer Relationship Management (CRM): The data can be used to personalize outreach and interactions with customers within your CRM system.
- Marketing Attribution: EasyInsights can help you understand how different marketing channels contribute to conversions and optimize your marketing spend accordingly.
Key Feature:
- Offers features like customer data platform (CDP) capabilities, marketing automation, and campaign management tools.
- Focuses on reverse ETL (extracting data from your warehouse and sending it to marketing tools) and offers pre-built integrations with various marketing platforms.
EasyInsights is a marketer’s preferred tool for first party data activation.
In this age of cross-channel marketing, EasyInsights offers a comprehensive platform to collect, store, and transform all your first-party data.
EasyInsights enhances marketing campaign efficiency in a cookieless landscape with accurate ad signals. It helps brands step away from surface-level metrics and unleash the potential of first-party data to optimize marketing strategies, ensuring a superior Return on Ad Spend (ROAS). It does all this, while being a highly affordable no-code platform with an exceptional customer support apparatus.
Sign up for a demo today to see EasyInsights in action.